Introduction
Item Response Theory (IRT) or Item Response Model is a new approach for Item Analysis to overcome classical test theory (CTT). It expands on the principles of ICCs by providing a comprehensive framework for understanding item and test performance.
According to Item Response Theory (IRT) approaches, each item on a test has its own item characteristic curve that describes the probability of getting each particular item right or wrong given the ability level of each test taker. With
Item Response Theory (IRT) developed in the 1950s and 1960s by Frederic Lord, Georg Rasch, Paul Lazarsfeld and other psychometricians (Lord, 1952; Lord & Novick, 1968) who had the goal of developing a method able to evaluate respondents without depending on the same items included in the test (Hambleton & Jodoin, 2003).
Now testers can make an ability judgment without subjecting the test taker to all of the test items.
Also known as Latent Trait Theory, Strong True Score Theory, or Modern Mental Test Theory.
Latent Trait:
This is the unobservable characteristic or ability that the test is designed to measure.
Examples include intelligence, math ability, or attitude towards a specific variable.
Represented by the Greek letter theta , ranging typically from -3 to +3.
Parameters of Item Response Theory (IRT)
IRT is grounded in the assumption that the probability of answering an item correctly is a function of the examinee’s latent trait and the item’s characteristics. These characteristics are quantified through three primary parameters:
1. Discrimination Parameter (a)-
The discrimination parameter evaluates how effectively an item distinguishes between test-takers of differing ability levels. A higher value means the item is sensitive to small ability differences, providing better differentiation.
2. Difficulty Parameter (b)–
The difficulty parameter identifies the latent ability level where half of the test-takers are expected to answer an item correctly. A higher value signifies a more challenging item, as only test-takers with above-average abilities are likely to succeed.
3. Guessing Parameter (c)–
The guessing parameter models the probability that a low-ability test-taker correctly answers an item by chance, such as through random selection in multiple-choice tests. This is crucial for evaluating item fairness and validity.
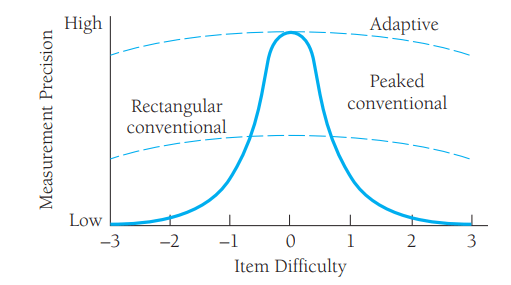
Item Difficulty
Models of Item Response Theory (IRT)
IRT models vary based on the number of parameters considered:
1PL Model (Rasch Model): Considers only difficulty ().
2PL Model: Considers difficulty (b) and discrimination (a).
3PL Model: Includes difficulty (b), discrimination (), and guessing (c).
Advantages of Using Item Response Theory (IRT)
According to Hayes (2000) IRT theory has many technical advantages.
- It based on traditional models of item analysis
- Can provide information on item functioning,
- The value of specific items,
- The reliability of a scale
IRT offers several advantages over classical test theory (CTT), including-
- Precision in Measurement- IRT provides item-level insights, enabling more accurate assessments of both items and examinees (Schneider et al., 2003).
- Enhanced Test Reliability- by focusing on well-functioning items, IRT reduces the effects of random guessing and other noise, increasing test reliability (Hayes, 2000).
- Ability-Level Scoring- unlike CTT, which bases scores on the total number of correct responses, IRT emphasizes the difficulty of items answered correctly, providing a more nuanced measure of ability (Smith et al., 2003).
- Adaptability- IRT facilitates computer-adaptive testing (CAT), where test items are tailored to the examinee’s ability, improving efficiency and reducing test-taking time (Schmidt & Embretson, 2003).
Applications of Item Response Theory (IRT)
IRT has broad applications across diverse fields-
- Educational Testing- used in standardized exams like the GRE and SAT to improve precision and fairness (Schmidt & Embretson, 2003).
- Psychological Assessments- measures constructs such as self-efficacy, anxiety, and personality traits with higher precision (Guion & Ironson, 1983). Test Development and Scoring:
- Develops tests where items are tailored to different levels of ability.
- Scores individuals more accurately than raw scores, considering item parameters.
- Health Metrics- used in patient-reported outcomes and quality-of-life assessments to ensure accurate measurement (Penfield, 2003a).
- Bias Detection- IRT helps identify items that function differently across demographic groups, aiding in the development of fairer tests (Sijtsma & Verweij, 1999).
Criticisms of Item Response Theory (IRT)
Despite its advantages, IRT is not without criticism-
- Complexity- IRT requires advanced statistical knowledge and computational tools, which may deter its adoption in traditional testing contexts (Hayes, 2000).
- Resource Demands- large sample sizes and extensive item banks are needed to implement IRT effectively (Guion & Ironson, 1983).
- Misinterpretation- misunderstanding IRT assumptions or parameters can lead to flawed test designs and inaccurate conclusions (Reise & Waller, 2003).
Conclusion
Item Response Theory IRT allows for the development of more reliable, valid, and adaptive tests. Despite its challenges, IRT’s benefits far outweigh its limitations, making it a cornerstone of modern psychometric research and practice.
The implications of IRT are profound. In fact, some people believe that IRT was the most important development in psychological testing in the second half of the 20th century. This theory has many technical advantages
References for Item Response Theory (IRT)
Aiken, L. R. (2008). Psychological Testing and Assessment. Boston: Allyn and Bacon.
Guion, R. M., & Ironson, G. H. (1983). “Item analysis and test construction.” Annual Review of Psychology, 34, 73-101.
Gregory, R. J. (2014). Psychological Testing: History, Principles, and Applications (7th ed.). Pearson.
Kaplan, R. M., & Saccuzzo, D. P. (2018). Psychological Testing: Principles, Applications, and Issues (9th ed.). Cengage Learning.
Hayes, J. R. (2000). The Complete Problem Solver. Philadelphia: Taylor & Francis.
Holland, P. W., & Hoskens, M. (2003). “Classical test theory and item response theory.” Handbook of Psychological Testing.
Meijer, R. R. (2003). “Computerized adaptive testing: Advances and challenges.” Applied Psychological Measurement, 27(3), 181-194.
Penfield, R. D. (2003a). “Examining the assumptions of IRT models.” Educational and Psychological Measurement, 63(6), 906-913.
Reise, S. P., & Waller, N. G. (2003). “How many IRT parameters are enough?” Psychological Methods, 8(2), 164-181.
Schmidt, F. L., & Embretson, S. E. (2003). “Modern measurement: The promise and pitfalls of IRT.” Psychological Science in the Public Interest, 4(2), 103-120.
Subscribe to Careershodh
Get the latest updates and insights.
Join 18,513 other subscribers!
Niwlikar, B. A. (2024, November 25). Item Response Theory (IRT); it’s 3 Parameters. Careershodh. https://www.careershodh.com/item-response-theory-irt-its-3-parameters/